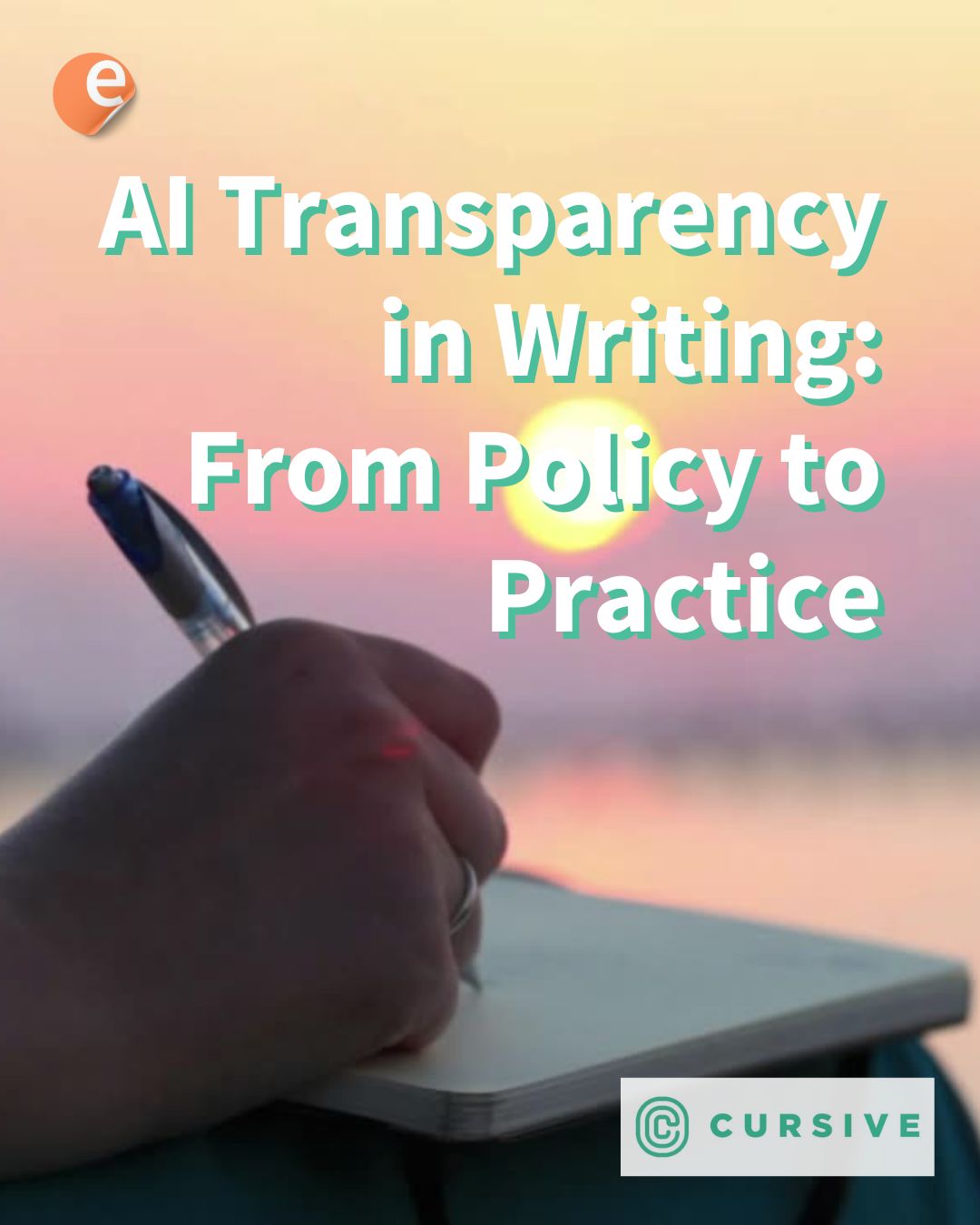
AI Transparency In Writing: From Policy To Practice
Consensus suggests we can no longer trust writing as an assessment. So why don’t we focus on writing as “practice” and ensure our students find value in their writing process?
Consensus suggests we can no longer trust writing as an assessment. So why don’t we focus on writing as “practice” and ensure our students find value in their writing process?
Could your school prohibit phone use for one day a week? This summertime campaign, just when people tend to spend the most time outside, could be extended through the school
More AI in Learning updates below For Charles Severance, Computer Science education should be about creativity and problem solving. More about deep understanding, less about syntax. Better known as Dr.
Canvas by Instructure is the most popular LMS in U.S. Higher Education today. But even if your organization is not a top LMS spender, you can still find out what all the fuss is about.
This article is written in collaboration with Ninja Tropic and eLearn Magazine. Let’s talk about microlearning in the context of your Learning Management System (LMS). Think of it as serving
In this article, we aim to shed light into the ways an admin is seen and how it should be instead, in order to bring a transformative understanding of the role and the LMS.
This article is written in collaboration with Ninja Tropic and eLearn Magazine. eLearning Professionals often picture the Learning Management System (LMS) at the core that powers learning experiences. But the
Disponible en Español The “Buyer Persona” quickly became one of the most popular and effective market research and planning tools of the decade, and it’s not hard to see why.
CSS is the visual language of the internet, and Learning Management Systems are no exception. In this list, we hope you find inspiration, simple ideas worth trying out, and perhaps
A small bounty of design goodness is hidden in plain sight on the forest that is the Moodle™ admin settings page. Check out the Theme Settings panel. Located at Site